WE ENABLE CUSTOMERS TO BUILD EXTRAORDINARY DEEP LEARNING BASED PRODUCTS
-min.png)
Technology
Embedl Model Optimization SDK revolutionizes the world of deep learning deployment by offering a cost-effective solution that not only saves on expensive hardware but also significantly reduces energy consumption. By harnessing the power of Embedl Model Optimization SDK, developers are empowered to accelerate the product development cycle, resulting in faster time-to-market and increased competitiveness.
Model Optimization SDK
Our groundbreaking Deep Learning Model Optimization SDK seamlessly integrates with popular deep learning development frameworks like Tensorflow and Pytorch, making it effortless to optimize models for maximum performance. With unparalleled support for a wide range of hardware targets, including CPUs, GPUs, FPGAs, and ASICs from renowned vendors such as Nvidia, ARM, Intel, STMicroelectronics, Texas Instruments, Qualcomm, and Xilinx, Embedl empowers developers to unlock the full potential of their deep learning projects.
-min.png)
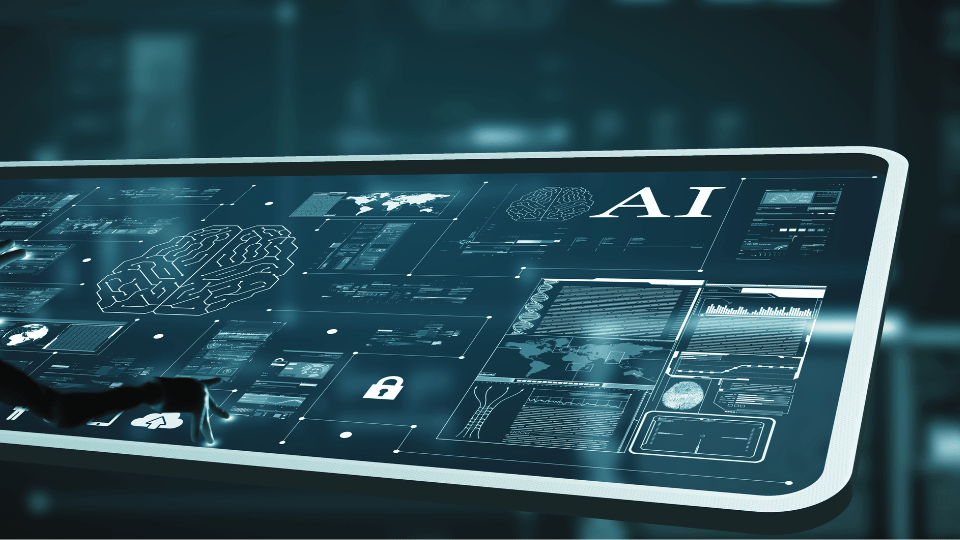
Research
Embedl is a spin-out from Chalmers University of Technology and research continues to be at the heart of what we do. We are exploring many different areas related to our mission of bringing DL to embedded systems. Topics include deep learning compression, network architecture search, quantization strategies and hardware design.
Your product could experience...
Up to 95% Reduced Memory Usage
Up to 83% Reduced Energy Consumption
Up to 18x Faster DL Inference on Device
Industries
Automotive
The automotive industry is known for its competitiveness and for being a cost-sensitive environment. Every company that wants to thrive in this market needs to be extremely careful with how they spend their resources. Embedl Model Optimization SDK integrates smoothly with popular automotive development frameworks and tools. Read more...
IoT
The Embedl Model Optimization SDK streamlines deep learning models for IoT devices, enhancing performance by addressing memory limits, reducing inference times, and cutting power use. Deploy superior applications in diverse industries and unleash your devices' full potential with Embedl. Read more...
Aerospace
Aerospace, represents an industry that places the utmost importance on the quality and performance of its components. Every material and component used in aerospace applications undergoes rigorous testing and certifications to ensure its reliability and safety. Our SDK helps ensure components meet stringent standards while accelerating development cycles. Read more...
What clients are saying
-
A great theme, wonderful design and very easy to customize. Also, their support is incredible - every question got answered fast and with quality solutions. Very recommended!
Jack Roy Digital Marketing Manager
-
A great theme, wonderful design and very easy to customize. Also, their support is incredible - every question got answered fast and with quality solutions. Very recommended!
Jack Roy Digital Marketing Manager
-
A great theme, wonderful design and very easy to customize. Also, their support is incredible - every question got answered fast and with quality solutions. Very recommended!
Jack Roy Digital Marketing Manager
-
A great theme, wonderful design and very easy to customize. Also, their support is incredible - every question got answered fast and with quality solutions. Very recommended!
Jack Roy Digital Marketing Manager
LinkedIn News
From our office
Our organization's success and dynamic culture are anchored by our employees. We value them for their unique skills, dedication, and the passion they bring every day!
From Cloud to Chip: Bringing LLMs to Edge Devices (Clone)
23 July, 2024Join our Upcoming Webinar: From Cloud to Chip: Bringing LLMs to Edge Devices. August 29th at 3 PM (GMT+2) Introduction t...
AutoTech: Detroit
3 June, 2024Meet Embedl at AutoTech: Detroit | 2024 June 5-6, 2024 Suburban Collection Showplace Novi, MI Don't miss our CPO Ola Tiv...
STARTUP AUTOBAHN EXPO2024
29 May, 2024June 6, 2024 Stuttgart, Germany At the STARTUP AUTOBAHN EXPO2024, we are thrilled to present a successfully completed pi...